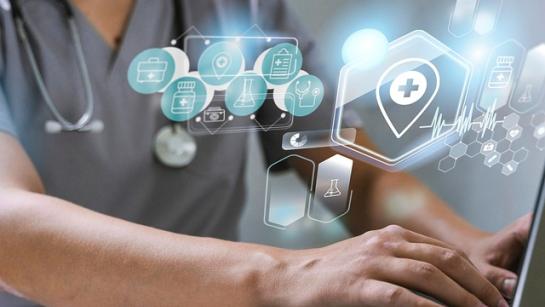
Jarosław Greser writes a guest blog-post on the legal possibilities and limitations of using synthetic data in the medical sector.
Introduction
The concept of synthetic data is of increasing interest to researchers and practitioners. This is leading to a growing number of practical applications. Some analyses suggest that by 2024, 60% of the data used to train AI algorithms will be synthetic data.
This raises the question of the legal possibilities of its use, especially for training algorithms that, by their characteristics, are subject to additional regulation. These include medical AI solutions, which can be classified as medical devices, both as a stand-alone algorithm and as a part necessary for the functioning of the device (Kiseleva 2020). At the same time, there are AI systems that have the characteristics of medical devices but are not included in this group because they have not been registered as such. There are no legal obligations to register device as a medical device, so the decision to go for this expensive and time-consuming procedure rests with the producers of such a device and is related with the business model chosen by them. Although the issues affecting such AI systems are the same as in cases of medical devices, their legal situation concerning the use of synthetic data will be quite different. I will not elaborate on this aspect in this article, but the existence of this problem needs to be highlighted.
What is synthetic data?
Synthetic data can be defined as artificially generated information that has analytical value. The main difference between it and real-world data is that synthetic data is not collected. This is due to the fact that this data does not exist and cannot be attributed to any individual. However, it has analytical value based on a statistical reflection of the real-world data set. Simply speaking, synthetic data mimics properties that are important from the user's perspective, without unnecessary information for the purpose of its use, which in many cases is personally identifiable information (PII).
The creation of synthetic data is well described in the literature (Nikolenko 2021) For the purposes of this article, it is worth recalling that Gal and Lynsky distinguish three methods (Gal, Lynskey 2023, p. 6-12). The first is based on transformations of collected data. For example, in the case of textual data such as medical records, this might involve deleting PII or changing the meaning by transforming the order of sentences or using synonyms. The second method is to produce synthetic data that reduces the need for collected data. This is done using a generative adversarial network (GAN) based solution where only one of the networks is trained on real data sets. The use of this method produces some of the best results in image generation, which can be used, for example, to train algorithms to analyse X-ray or MRI images. The third type are methods without direct use of data, based on the use of a simulator that generates synthetic data based on a set of rules that determine the relationships between the relevant data attributes. Such data can be used, for example, to test systems or for medical training.
Synthetic data and Medical AI
Proponents of using synthetic data to train medical AI systems point to many benefits. The first is much lower cost of data acquisition, which includes reducing the costs of cleaning, labelling, and organising raw data, as well as the costs associated with accessing patients.
The second area is the ability to augment existing real-world datasets. This is particularly important in the case of rare or orphan diseases, where the ability to obtain a diverse study sample is limited. This is especially true for disadvantaged or discriminated groups. This phenomenon has long been highlighted in the context of drug testing (Dresser 1992), but it also applies to medical devices and is part of a wider trend to avoid bias in AI algorithms. Data augmentation can also include the creation of virtual twins. This is one of the tools of personalised medicine, in which a patient's medical profile (including genetic profile) is digitally mirrored and various simulations are run of, for example, the response to specific drugs that the patient might hypothetically be taking (Venkatesh, Raza, Kvedar 2022). There are also benefits in terms of saving energy and storage space, overcoming the barriers associated with transferring data between organisations, and improving the quality of test data.
At the same time, many limitations of synthetic data are highlighted. Accuracy risks are among them. These include the general data management principle of 'garbage in - garbage out', namely the risk of duplicate bias or errors if these are present in the database from which the data were generated. A specific challenge for the medical sector is the question of the statistical relevance of synthetic data. Borderline cases may be omitted as not relevant to the model. For example, rare or ultra-rare diseases, newly discovered diseases or non-standardised symptoms of known diseases may be excluded from analyses. In addition, current models are ineffective at capturing and processing context. It is important to note that medical records, especially for mental health, can contain a lot of information, the correct interpretation of which requires an understanding of cultural context or language codes (Ive 2022).
In addition, various challenges are identified related to the lack of standards for the creation of synthetic data, the impact of their use on the explainability of the algorithm, or the change in power relations associated with the strengthening of the market advantage of those with large data sets Gal, Lynskey 2023, p. 19-28).
Privacy is a separate issue. In materials on synthetic data, one can often find claims that it is an effective technique for preserving privacy, or even suggestions that the use of synthetic data has not resulted in a GDPR penalty. It is important to stress that such general statements can be misleading. Research shows that such a claim can be true, but only in specific cases and when additional conditions are met (Ive 2022). At the same time, maintaining a high level of privacy has a significant impact on the usefulness of the data, especially in terms of transparency (Stadler, Oprisanu, Troncoso 2022). It can therefore be assumed that in the case of synthetic data for medical training it requires a very careful analysis of the level of privacy that the collection provides and the risk of violating the rights of the individuals whose data were used to create the dataset.
Synthetic data and the possibility of training medical AI in MDR
The cornerstone of medical device regulation in the European Union is the Medical Devices Framework, which consists of the Medical Devices Regulation [MDR] and the In Vitro Medical Devices Regulation [IVDR]. I will focus on the analysis of the MDR, but it can be assumed that the conclusions are also applicable to the IVDR, as both acts are based on the same assumptions.
The MDR does not contain specific provisions on the training of AI systems, let alone synthetic data. At the same time, Article 5(2) establishes the principle that each device must meet the general safety and performance requirements set out in Annex I that apply to it, taking into account its intended purpose. This principle is complemented by the provision in Annex 1, point 17. It states that electronically programmable systems, which in the context of the MDR includes AI systems, shall be designed and manufactured in accordance with the state of the art. The safety and performance of AI systems is directly related to the data used to train them. And the current state of the art does not allow the assumption that the use of synthetic data will lead to better results than the use of real data sets. Their use should therefore be preceded by a comprehensive analysis of the risks and an evaluation of the benefits and risks from a patient safety perspective.
Synthetic data may also be used as validation or test data. At the same time, the data used for these purposes falls into the scope of definition of clinical data in Article 2(48) of the MDR, i.e. information on safety or performance generated by the use of a device. At the same time, this provision indicates a closed catalogue of sources of clinical data, which does not mention synthetic data. It would appear that data from 'clinical investigations' would not cover synthetic data, as the definition of this term indicates that 'one or more human subjects' shall be involved. Perhaps synthetic data could be used as part of the clinically relevant information from post-market surveillance, but this would require a very strong case to be made.
Summary
The applicability of synthetic data in medical AI training may also be affected by other legislation, in particular, the AI Act and the European Health Data Space. In the first case, specific rules on the quality of training data or technical documentation may apply. In the second case, it will cover the use of synthetic data as secondary data. Both Commission’s proposal are in the legislative process, and depending on the outcome of this procedure, their adoption may change a lot in using synthetic data. Among the existing provisions, the GDPR seems to be the most important. Taking into account the state of the art, it can be assumed that in most cases the synthetic data will not be anonymised and therefore it will be necessary to comply with the requirements imposed by this act.
References:
1. Anastasiya Kiseleva, AI as a Medical Device: Is It Enough to Ensure Performance Transparency and Accountability in Healthcare? European Pharmaceutical Law Review, 1/2020;
2. Michal Gal, Orla Lynskey, Synthetic Data: Legal Implications of the Data-Generation Revolution, LSE Legal Studies Working Paper No. 6/2023;
3. Sergey I. Nikolenko, Synthetic Data for Deep Learning, Springer 2021;
4. Rebeca Dresser, Wanted. Hastings Center Report, vol. 22, 1992
5. Kaushik P. Venkatesh, Marium M. Raza, Joseph C. Kvedar, Health digital twins as tools for precision medicine: Considerations for computation, implementation, and regulation, npj Digital Medicine 5/2022
6. Julia Ive, Leveraging the potential of synthetic text for AI in mental healthcare, Frontiers Digital Health, Sec. Digital Mental Health 4/2022
7. Theresa Stadler, Bristena Oprisanu, Carmela Troncoso, Synthetic Data – Anonymisation Groundhog Day, https://www.usenix.org/conference/usenixsecurity22/presentation/stadler